What makes students and instructors choose, sustain their learning with, and learn meaningfully from instructional videos?
I recently became the homeowner of a mid-80s colonial revival and have taken on several projects to bring it out of the 80s and into a more contemporary style. Levelling out a sunken dining room is much more challenging than I expected. While struggling with this and other projects, I have often stopped and thought, “I need to YouTube this.” Whether it be during the completion of a home project, cooking a new recipe, planning an international trip, or completing an academic task – I know I am not alone in reaching for my phone to open a video platform, like YouTube, to find the answer to my problem from someone, who I assume, is more expert than myself.
As an instructor for courses on the psychology of learning, cognition, and development, I also search for videos where someone more expert or clearer than myself can explain a complex topic. For example, I like to use this TEDx Talk by Dr. Courtney Griffin when teaching my students epigenetics (see the video below).
TEDx Talk by Dr. Courtney Griffin on epigenetics.
I like Dr. Griffin’s talk because I know she used advanced self-regulated processes to develop her talk according to the goal of clearly and effectively communicating a complex topic to an audience that likely varied in their prior knowledge of that topic, aka. epigenetics. I feel confident Dr. Griffin could and did use these advanced self-regulated processes to prepare her talk because she is the Vice President of Research and Professor in the Cardiovascular Biology Research Program at the University of Oklahoma Health Sciences Center. In other words, Dr. Griffin is an expert, and experts can use their expansive prior knowledge to perform complex, higher-order cognitive processes – like planning, developing, and presenting a clear talk on a complex topic.
Fortunately, my expertise in multimedia learning prepared me for vetting the efficacy of this video when I was developing my course. But what criteria do I use to vet the efficacy of a video on DIY home projects, of which I have no prior knowledge? What criteria do students use to vet the efficacy of a video on photosynthesis when completing their biology homework? Or what criteria does a sixth-grade history teacher use to vet the efficacy of a video on the ancient cultures of Greece? Using evidence-based criteria in each scenario is vital for choosing and learning effectively from an instructional video.
Design criteria supported by multimedia learning
Research on the cognitive theory of multimedia learning provides us with a valuable set of criteria for understanding how instructional videos support learning. According to this theory, we process verbal (e.g., the oral narration in a video) and visual (e.g., the images, graphics, or visual of the presenter in a video) information across dual channels in our working memory, and each of these channels has a highly limited processing capacity.
To take full advantage of the limited processing capacity in the verbal and visual channels, we must successfully select relevant information from the video, organize both the verbal and visual information into a meaningful mental model, and integrate those models as well as with our relevant prior knowledge.
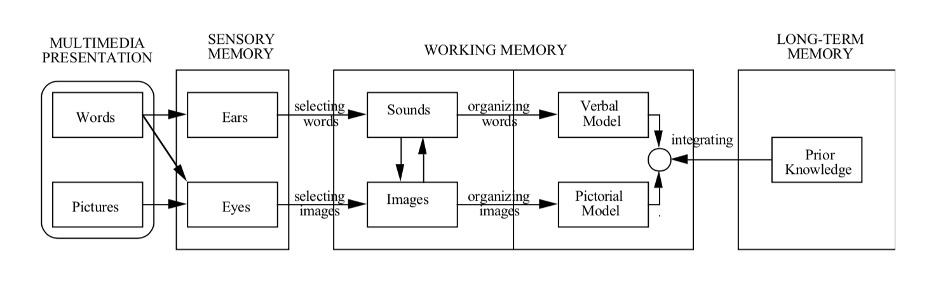
After decades of research on the cognitive theory of multimedia learning, several design principles that support learning from videos have emerged that (1) limit cognitive processing that is irrelevant to the learning goal, (2) help to manage essential cognitive processing, or (3) foster generative cognitive processing that is necessary for meaningful learning. Putting each of these design principles together gives us initial criteria for vetting the efficacy of instructional videos.
Let’s go back to a previous example – a student searching for a video on photosynthesis. According to design criteria from the cognitive theory of multimedia learning, the student should search for a video that pictorially shows the phases of photosynthesis while a voice orally narrates the processes that correspond with each picture (i.e., redundancy principle and temporal contiguity principle). In addition, the student should be able to pause and rewind the video to match their processing speed (i.e., the segmenting principle), and the video should incorporate practice questions at the end of important segments to help the student test themselves on what they have learned (i.e., generative activity principle). These principles help limit cognitive processing irrelevant to the learning goal, manage essential cognitive processing, and foster generative cognitive processing, but some questions remain.
For example:
Are students pausing instructional videos to answer embedded practice questions?
Or, what if a student doesn’t pause and rewind the video to match their comprehension speed and, instead, just watches the whole video at 2x speed to get through it?
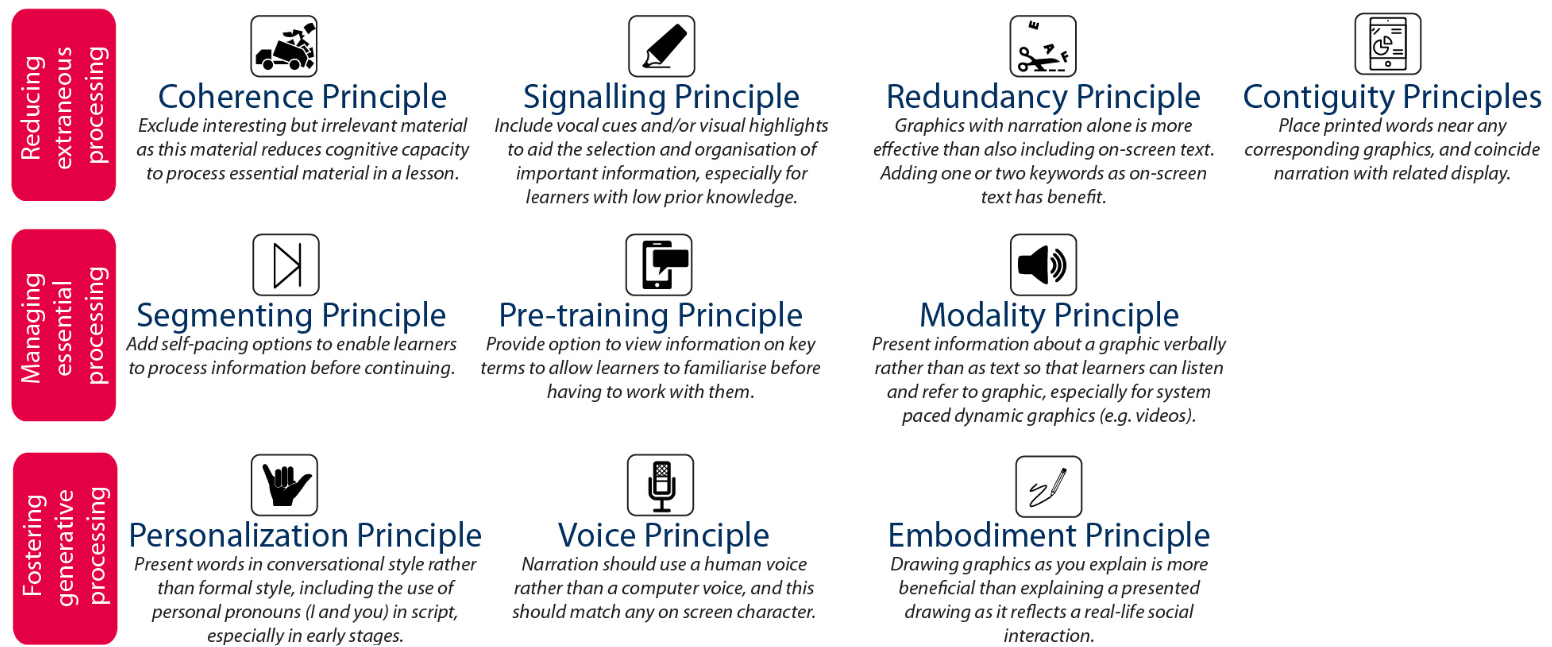
Evidence-based designs do not guarantee deep engagement with videos
My colleagues at the University of North Carolina at Chapel Hill and I conducted a large-scale classroom study with undergraduate biology students where we surveyed them at the beginning of the semester about various learning processes, such as their typical strategy use, self-efficacy, and achievement goals for the course. Learners can be motivated by three primary types of achievement goals, (1) the goal to learn meaningfully and increase one’s skills (mastery approach goals), (2) the goal to outperform peers (performance approach goals), and (3) the goal to not look incompetent in front of peers (performance-avoidance goals). Then, we collected all the digital trace data students generated throughout the semester. Digital trace data is recorded each time a learner clicks on a digital tool in their course learning management system or other educational web tools. One such digital tool was the platform through which instructional videos for the course were hosted.
In a recent study (Kuhlmann et al., 2023), we tested how students’ achievement goals predicted how long they watched course videos and how they performed on practice questions they were supposed to answer in between watching the videos (i.e., making use of the generative activity principle from the cognitive theory of multimedia learning). We found only students’ mastery goals to be related to better performance on practice questions in between videos and, in turn, better performance on the course exam. We were surprised to find that no achievement goals were related to how much time students spent watching the videos. This finding led me to wonder – perhaps meaningful learning from videos comes from a deeper form of engagement than simply spending the time to watch the full video.
To understand deeper types of engagement with videos, we used sequence mining to explore the order and frequency at which students use video player tools. Sequence mining allows researchers to get a meaningful string of events from a large dataset of digital traces that occur commonly among students in the sample. For example, a student watching a video in their biology course might:
- Play the video for 1 minute,
- Pause the video for 30 seconds to write a note,
- Continue playing the video for 2 minutes,
- Pause the video to answer an embedded practice question,
- Realize they don’t know the answer to the question and rewind to 1:12 minutes to review that segment.
If this string of video-watching behaviors is common among many students in the course, it is identified in the sequence mining analysis, and the frequency of its occurrences is counted per student.
The sequence mining analysis uncovered four common categories of video-watching behaviors: repeated scrubbing, speed watching, extended scrubbing, and rewinding. Repeated scrubbing occurred when students had quick and frequent bursts of fast-forward behaviors. Speed watching occurred when students increased the playback speed of the video. Extended scrubbing occurred when students had infrequent and drawn-out fast-forward behaviors. Rewinding behaviors occurred when students rewound the video.
When we analyzed how each type of video-watching behavior interacted with students’ prior knowledge and how that interaction was related to course exam performance, we found something surprising – the increased use of rewinding and speed-watching behaviors was related to better exam performance, but only for students with lower prior knowledge. It makes sense that rewinding and reviewing missed or confusing content helps students with lower prior knowledge; however, the same finding for speed-watching seems surprising.
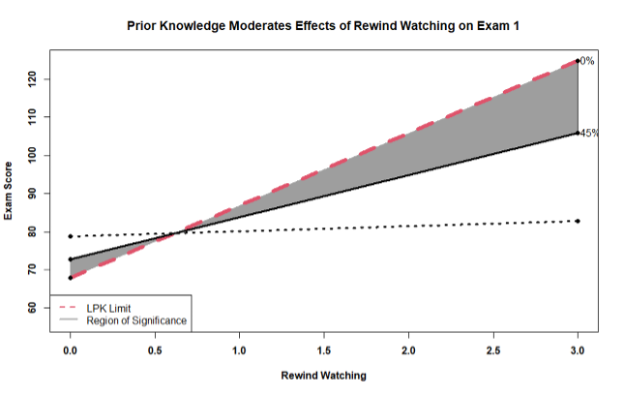
Yet, this finding aligns with the expertise reversal effect, which occurs when an instructional format that is beneficial for students with lower prior knowledge loses its advantage for students with higher prior knowledge. Students with higher prior knowledge might have found the content and/or the instructional scaffolding in the videos to be redundant, which led them to speed-watch the videos and skip content that would have been meaningful for their exam performance. However, students with lower prior knowledge may have found the content and/or instructional format of the video to be helpful, and therefore primarily engaged in speed-watching behaviors to efficiently allocate their cognitive effort while maintaining sufficient engagement for learning. Another potential explanation for these findings is that higher prior knowledge students were speed-watching because they overestimated what they knew and, therefore, skipped necessary information that hampered their exam performance. This suggests higher prior knowledge students might also experience expertise reversal effects due to poorer self-regulation of their learning, like a miscalibration between judgments of their own knowledge and actual knowledge, which leads them to disengage from productive behaviors.
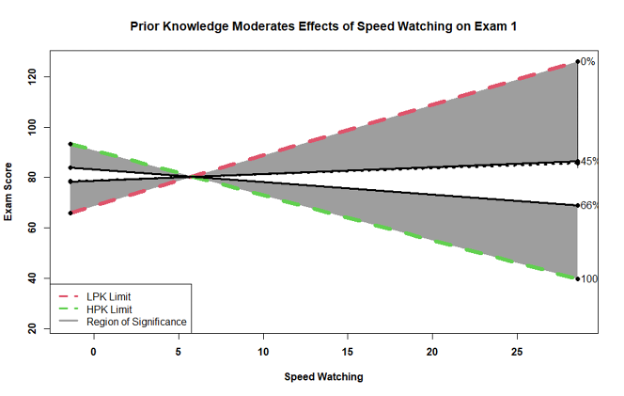
Setting criteria that includes evidence-based design and fosters engagement
Findings from both studies build on design recommendations previously supported by the cognitive theory of multimedia learning, such as the redundancy, segmenting, and generative activity principles. Students and instructors should consider the varying motivations one has to watch videos and complete adjunct activities. For example, adding credit or performance components to these activities or using other generative activities, like teaching a peer or explaining to oneself, might be more likely to motivate students with both mastery and performance goals. Also, just watching the course videos might not be enough to learn meaningfully from them. Instructors should consider how they can help students engage in the right video-watching behaviors, at the right time. For example, instructors might consider pre-training students on productive video-watching behaviors and highlighting key moments for engagement with video content. In the future, I hope to see video hosting software include more adaptive and intelligent features that promote generative activity, such as embedded pedagogical agents that listen to student-generated explanations and provide real-time feedback.
Going through and recovering from a pandemic has certainly emphasized the popularity and power of learning from videos. I hope this article helps many of you think about the criteria by which you choose to watch a video for learning and, maybe even join me in the search to better understand, identify, and share effective criteria with teachers and students.