“We all sometimes feel that we make different decisions when we’re hungry. We can now be more specific about this, by distinguishing planned vs. habitual actions.” – Sanjay Manohar
Hunger is a beast. It can make us act unlike ourselves at times. As every Snickers ad reminds us: you’re not you when you’re hungry.
We know that hunger can have a negative influence on cognitive processes such as attention or learning, but van Swieten and colleagues show us that hunger can also lead us to increase reinforcement-driven decision-making.
In their paper in the Psychonomic Society journal Cognitive, Affective & Behavioral Neuroscience, Maaike M.H. van Swieten, Rafal Bogacz, and Sanjay G. Manohar (pictured below) investigated the effects of hunger on a decision-making task. They asked participants to come in for two visits: one where they had a normal day of eating breakfast before their study visit, and another where they had to fast from 8:00 pm the night before. Nobody likes fasting, but we applaud these brave folks for doing it in the name of science. All participants completed both conditions in a random order, which made for a great within-subjects design.
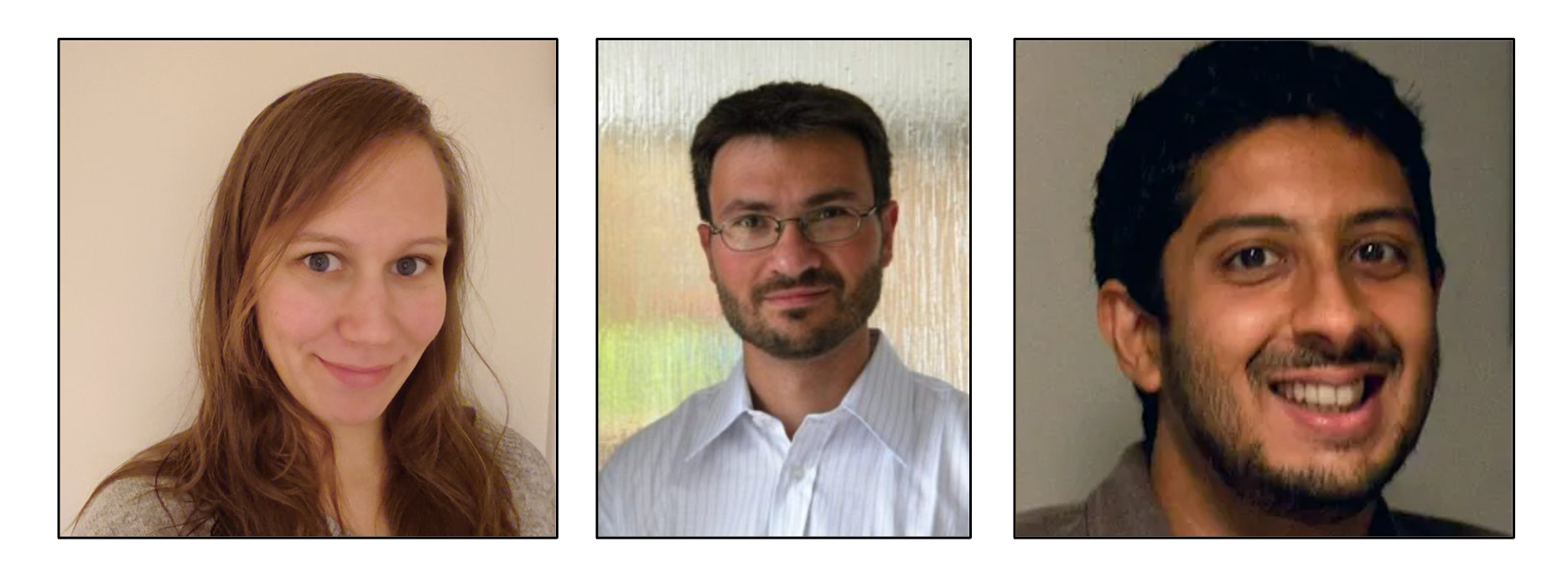
I’m no expert on the science behind hunger, but I know that when I get really hungry (even hangry), I will do anything for food. In that sense, the biological drive for food makes eating a primitive reinforcement system. We get hungry, we eat, mission accomplished. In that state of hunger, we seek the reward of food. In the current study, the authors asked if hunger triggers other reward-driven behavior—not simply food-seeking behavior.
To test this, van Swieten, Bogacz, and Manohar asked participants to complete a decision-making task, in which the reward was monetary, not food-related. Decision-making, in general, can be structured around a model-based planning system, which uses “knowledge about how actions change the state of the world”—or a primitive model-free system, which uses “memory only about how rewarding actions were in particular states.” The model-based system uses logic to determine the best course of action, while the model-free system is impulsive based on recent rewards.
The task in this study was a game where participants had to make two decisions. First, they had to select one of two rockets. The chosen rocket transported them to a planet (in a galaxy far, far away). Next, they had to select one of two aliens. If they selected the correct alien for that trial, they received a monetary reward. This design is known as the Daw two-step task, and it has been used in many similar sequential decision-making paradigms.
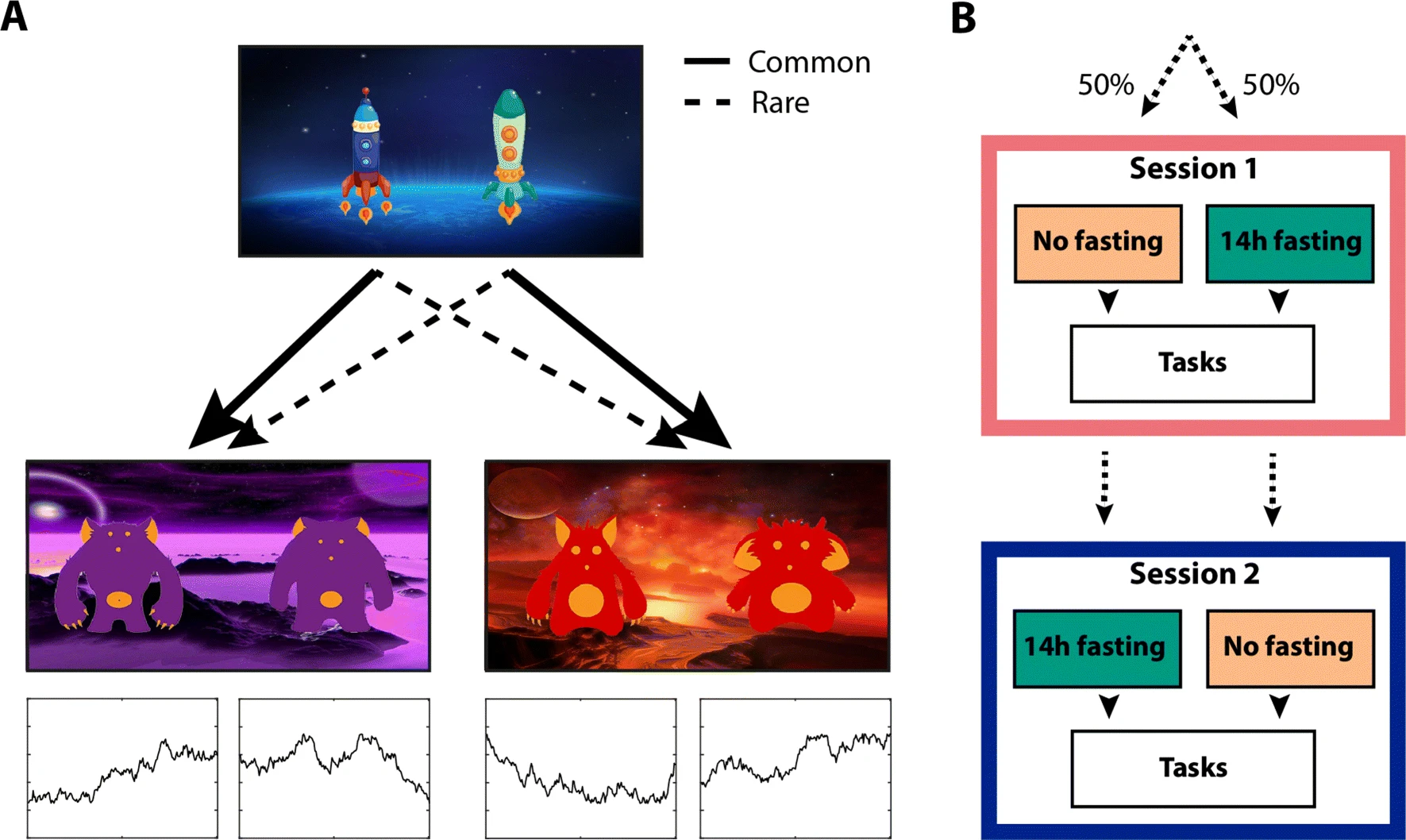
Here’s the catch: the probability of obtaining a reward changes throughout the task. Each rocket will predictably go to the same planet 70% of the time, but it will also land on the other planet 30% of the time. The aliens will then deliver a reward with fluctuating probabilities between 25% and 75% based on a Gaussian random walk.
If you win the alien money, you’ll want to return to that planet in the next trial to win more! This is where the decision-making comes in. In a model-based system, you will be tracking which rocket typically goes to which planet, and you’ll select the rocket that normally goes to the money planet. However, let’s say you won the money in a “rare transition” where the rocket landed on the planet it doesn’t normally visit. If you use the model-free approach, you would still select that rocket in the next trial because it was rewarding (and you don’t care about where it normally goes). The model-based system tries to learn and follow the typical pattern of events, and the model-free system simply goes where the money was in the last trial.
Over the course of 201 trials, the researchers used a one-trial-back stay-switch analysis to quantify each participant’s pattern of staying with the rocket from the last trial or switching to the other rocket. If you’re interested in probabilistic statistics and computational modeling, I encourage you to read this paper for more details on the calculations. Based on this analysis, they could determine if someone was making model-based or model-free decisions.
It turns out that when people were hungry, they made model-free choices more frequently. Furthermore, hungry people actually performed better on the task and earned more alien money! The model-based system did not change significantly as a function of hunger. This suggests that hunger increases a person’s reliance on primitive reinforcement mechanisms. Even though food wasn’t involved in this task, participants increased simple reflexive decision-making when deprived of food.
Who said you can’t teach a hungry dog new tricks? This study shows that hunger improved learning and the effect that a reward has on a hungry person. The downside is this reflexive impulsivity could get us into trouble at times. I suppose the lesson to be learned here is: don’t make any life-changing decisions until you’ve had a Snickers.
Featured Psychonomic Society article
van Swieten, M. M. H., Bogacz, R., & Manohar, S. G. (2021). Hunger improves reinforcement-driven but not planned action. Cognitive, Affective & Behavioral Neuroscience, 21:1196–1206. https://doi.org/10.3758/s13415-021-00921-w