Picture yourself sitting in front of a seemingly endless stack of exams to grade, full of open-ended questions, with responses demonstrating varying levels of understanding. Now imagine your joy when you flip open an exam with a first response that just gets it. An exceptional answer that demonstrates a true mastery of the material. Did you accidentally leave the answer key in your pile? You read on to the second question and notice it doesn’t quite fit with the key. A few pieces of the answer aren’t present. But you give the student the benefit of the doubt and mark it right, figuring they probably knew the answer, but maybe they just didn’t word it perfectly. You mark it with an immediate A+ and sit back, content that at least one student understood your lectures for the “Cognitive Biases and Heuristics” section of the course.
This scenario illustrates the halo effect, which is a way that human reasoning shows bias. The halo effect refers to the tendency of initial impressions to influence subsequent judgments, meaning that when you make a positive assessment, your next assessment will likely be more positive than it would have been otherwise. Knowing how well the student did on question #1 systematically affects how well you think they did on question #2. But what if you had seen question #2 before question #1? You might view the same responses in a different way, leading to a different grade depending only on how the questions were shuffled. In this light, the halo effect may seem like an example of how human reasoning is irrational, incorporating arbitrary factors into critical decisions. As a result, scholars have long suggested that biases like the halo effect occur because the human mind uses heuristics, or mental shortcuts, to make complex decisions without using too many cognitive resources.
In a recent paper in Cognitive, Affective, & Behavioral Neuroscience, researchers Paul Sharp, Isaac Fradkin, and Eran Eldar (pictured below) provide an alternative to this long-held interpretation of cognitive biases. Rather than illustrating how humans try to simplify the complexity of the computations needed to make a decision, the authors argue these biases may instead reflect a quite complex process of hierarchical inference.
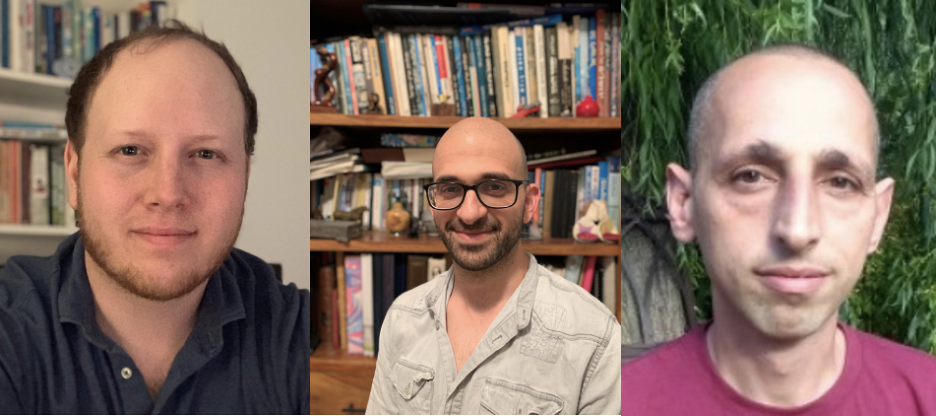
Hierarchical inference involves gathering information and making inferences from multiple sources from multiple levels. For example, in the exam grading scenario, there are two levels of information available to you when grading a question: the specific content of their response to that question and your view of the student’s overall level of knowledge on the topic (which may be informed by how well the student did on the previous question). Using simple, nonhierarchical inference would lead you to believe that students’ knowledge of each individual question is unrelated to their knowledge of any other question. However, using hierarchical inference, you estimate their actual knowledge by incorporating information about the student’s typical knowledge, which is updated after seeing their response to the first question, then their response to the second question, and so on. This inference process, depicted in the image below, leads to a less noisy estimate of the student’s actual knowledge, a more informed decision and less uncertainty in the grade.
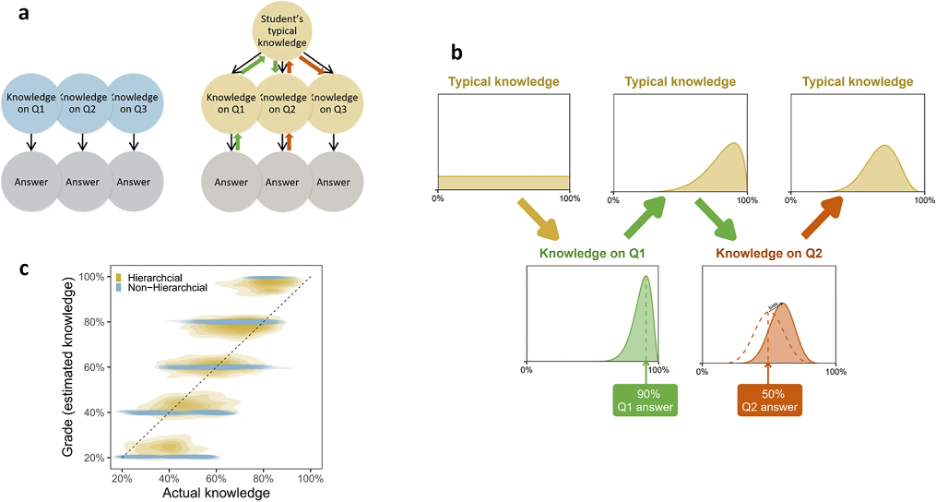
The authors reinterpret other cognitive biases, such as affective evaluation bias, the hot hand illusion, and anchoring as hierarchical inference, illustrated below. Further, hierarchical inference is involved not only in decision-making, but can also be seen in other cognitive processes, like language and perception. According to the authors,
“Perceptual neuroscience, for example, has shown how higher-level inference about the general context portrayed by a visual stimulus (e.g., ‘I believe I’m looking at a picture of a typical day in a city’) shapes lower-level inferences about individual objects within this context (e.g., ‘this blurry image must be a car parked next to a sidewalk’).”
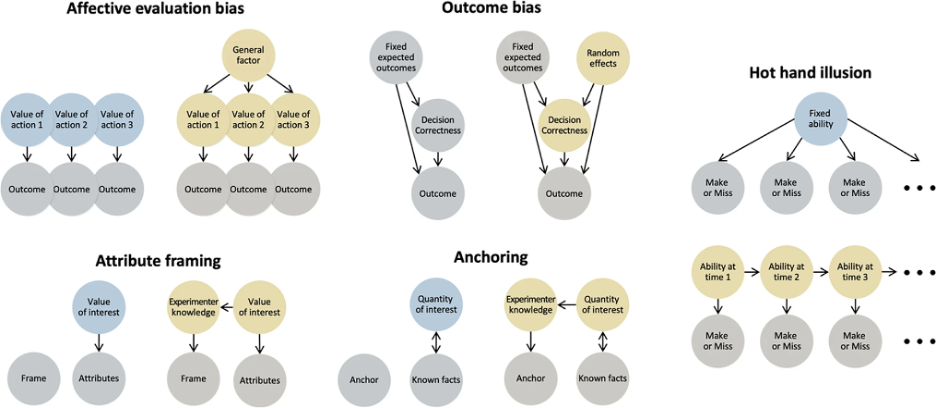
The alternative view of bias proposed by the authors has important implications for how humans make decisions. For instance, under the traditional view, biases occur when people simplify decisions and use heuristics that minimize the computations needed to arrive at an answer. Thus, biases should most strongly emerge when people are spending little effort on the decision at hand and making quick decisions. By contrast, if, as the authors suggest, biases arise from more complex processes of hierarchical inference that incorporate a variety of sources of information, then we would expect the exact opposite: people should be most biased when they are putting more effort and computational power into solving a problem.
The exact sources of bias are likely to vary on a case-by-case basis. Biases may not always emerge from simplifying heuristics, but they may not always emerge from complex hierarchical inference either. As the authors put it,
“Ultimately, we believe a complementary set of ideas is needed to comprehensively address the diverse set of cognitive biases, including not only hierarchical inference and resource rationality, but also evolutionary suboptimality and motivated cognition (Williams, 2020). Determining what explanation, or combination of explanations, best suits each instance of a bias requires careful case-by-case study, which we hope the present paper will motivate and inspire.”
So, the next time you find yourself grading an exam question, letting the previous question’s answer guide your judgment, consider that you might not just be taking a mental shortcut to get through the stack quicker–you might actually be conducting a complex set of computations to make your evaluation more informed and less uncertain.
Featured Psychonomic Article
Sharp, P. B., Fradkin, I., & Eldar, E. (2022). Hierarchical inference as a source of human biases. Cognitive, Affective, & Behavioral Neuroscience, 1-15. https://doi.org/10.3758/s13415-022-01020-0