The world around us is structured. Certain features of objects just go together. Imagine a muscular sports car in a 1950s black-and-white photograph, like this classic Ferrari 340:
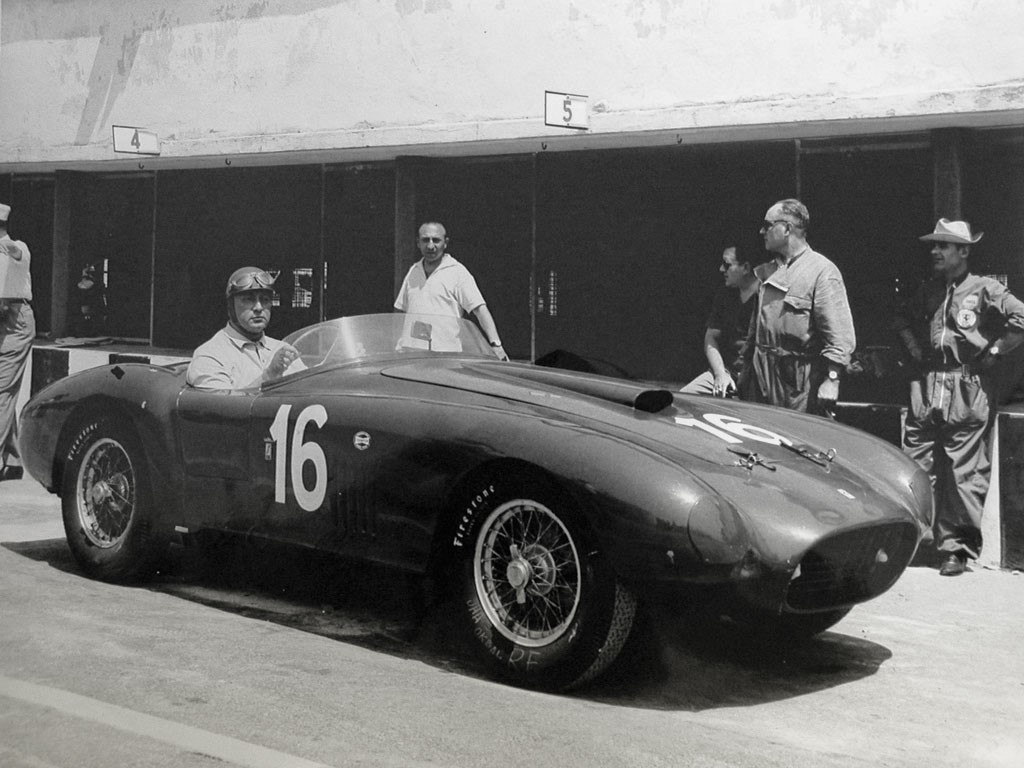
What do you think its color was?
And what color would you guess a 1950’s VW beetle in a grayscale image was?
Chances are you did not think that the Ferrari was some mushy Khaki and that the VW beetle was fire-engine red. On the contrary, the Ferrari pretty much had to be red. The beetle also had a color but that one was perhaps best described as “dull”.
This example represents one instance of “feature inference”, which is the process by which we predict inexperienced features of objects on the basis of their category. We know that Ferraris are red whereas old VW beetles reside in a region of color space largely uninhabited by other artifacts. Similarly, we know that small birds are more likely to sing than large birds, and if we find a piece of furniture with springs in it we may well surmise that it is meant for sitting or sleeping on.
But what happens when we are uncertain about the category of the object in question? What if we were unsure whether the first picture above was really a Ferrari—what if it was a British Lotus instead? Ferraris are mostly red, but traditionally, British race cars were liveried in British racing green. Do we think the color might be some mix of green and red, such as yellow (for additive mixing)? Or do we consider the color to be “30% red+70% blue”, based on our estimated probabilities of the car’s identify?
One highly influential model of this process, proposed by John Anderson, is known as the rational model. The model stipulates that people infer the probability of an object’s category membership from its known features, and then infer the overall probability of the inexperienced feature by summing the constituent probabilities across all candidate categories. To illustrate, suppose a Ferrari is always red (which was true in the 1950s) whereas a Lotus has a small chance (say 10%) of also being red, then the probability of a perfectly ambiguous car (i.e., 50/50 chance of being a Ferrari or Lotus) being red is thought to be 55% (1.0 x 0.5 + 0.1 x 0.5). An important attribute of the rational model is that people are thought to consider all candidate categories when making inferences about inexperienced features of objects.
How well does the rational model predict people’s responses in such inference tasks?
A recent article in the Psychonomic Bulletin & Review addressed this question by reviewing the existing evidence and by presenting several new experimental tests. Researchers Elizaveta Konovalova and Gaël Le Mens first examined the existing evidence base and noted that this literature had converged on the consensus that the rational model overall provided a poor fit of the experimental data.
Konovalova and Le Mens then pinpointed the reason for this failure. Central to the model is the assumption that within-category feature correlations are exactly zero—an assumption known as conditional independence. This assumption, however, was violated in all of the experiments published to date that examined how people inferred the missing features of objects.
The question then arises, how well would the rational model predict people’s performance in a task environment in which conditional independence actually holds? To create this environment, Konovalova and Le Mens created a category structure based on continuously-valued (rather than discrete) features. The figure below shows this space, in which stimuli were categorized as either “mice” or “rats” based on the values of two fictitious hormones (Rexin and Protropin). This category space instantiates conditional independence because given that an animal is a mouse (or a rat) there is no correlation between the values of the two hormones.
In the experiment, people were presented with a value of Rexin and were asked to predict the level of Protropin (without being explicitly told what the animal was). Note that because the values of Rexin partially overlap between the two categories, the categorization of an animal based on Rexin alone must remain probabilistic in many cases.
The figure below shows the predictions of the rational model for this task. The model suggests that in the central region of ambiguity, people would propose one or the other level of Protropin (55 or 75) with a probability equal to the probability of category membership based on the level of Rexin.
The next figure shows the data from a subset of participants. In each panel, the response (level of Protropin on the Y-axis) is shown as a function of the level of Rexin for the stimulus. Recall that participants neither knew nor had to indicate the category membership of the stimulus. Of greatest interest here are the “oscillations” in the central region around Rexin values of 65. These oscillations confirm that participants return the value associated with one or the other category, depending on how they categorized each particular test stimulus.
Konovalova and Le Mens explored the results by comparing the ability of the rational model to handle the data to two other candidate models (their details are not relevant here). That comparison unequivocally favored the rational model. By and large, people’s responses across 4 experiments using this category space conformed to the expectations of the rational model.
What can we learn from these results?
The most striking feature of the results reported by Konovalova and Le Mens is that people did not average the two plausible values of Protropin (75 and 55). Instead, they reported one or the other but nothing in between. This strongly implies that people did not integrate across the two categories at the decision stage—they did not turn a bit of Ferrari-red and Lotus-green into a yellow compromise. Instead, they classified each stimulus probabilistically and then committed themselves to the associated value of Protropin. Nonetheless, people considered both categories when making their decisions because their (tacit) choice of category was sensitive to the relative likelihood that a given value of Rexin came from a mouse or a rat.
Turning to the theoretical bottom line: The rational model proposed by Anderson is a good predictor of people’s performance in an environment in which conditional independence holds.
Psychonomics article highlighted in this post:
Konovalova, E., & Le Mens, G. (2017). Feature inference with uncertain categorization: Re-assessing Anderson’s rational model. Psychonomic Bulletin & Review. DOI: 10.3758/s13423-017-1372-y.